2021 must-know data market trends
2021 must-know data market trends
We know hiring is hard… If you want to get ahead of data market trends to maximise your potential, read more!
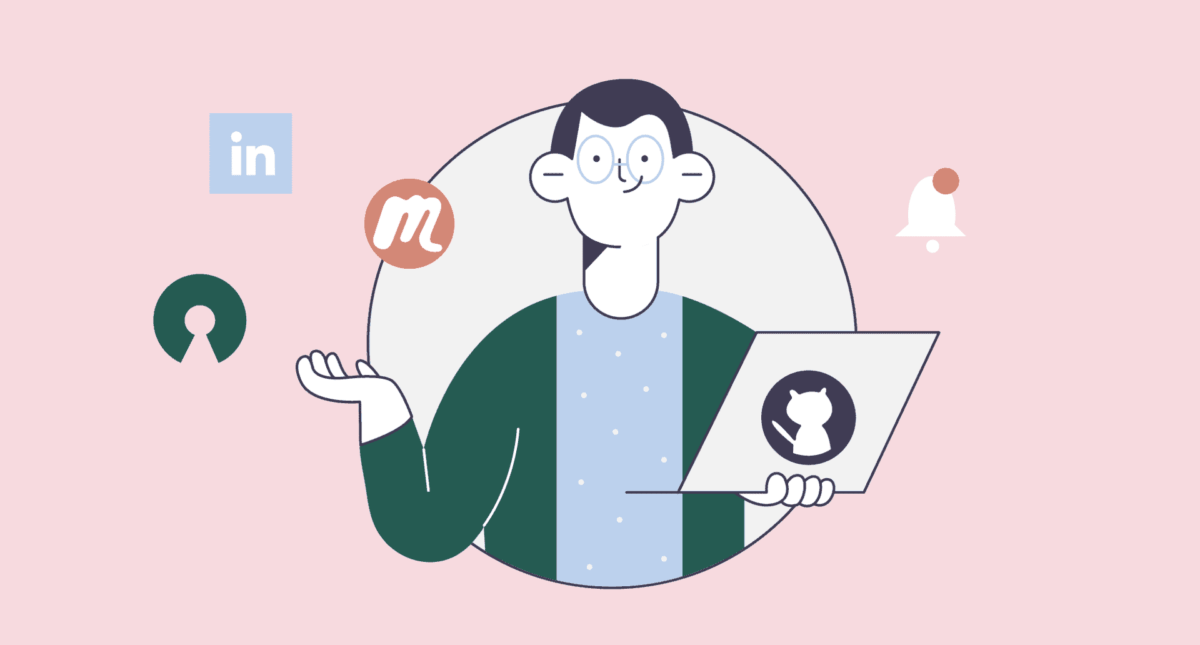
This year has been a wild one in the recruitment world. A collection of influences including, lagged hiring from 2020, unexpected business performance and remote working has led to one of the more competitive markets in recent memory.
So… we know hiring is hard. Where should you, as a data specialist, be focussing your learning time and training budget in order to maximise your potential at the moment?
Companies struggle to define in a title what they are actually expecting (do you really need a Data Scientist, or is it a Data Engineer in disguise?). Generally, data teams are being split into Data Science (applied machine learning); Data Engineering (warehousing, infrastructure and cloud) and Data Analytics (dashboarding, visualisation, domain knowledge).
But what skills and subsections are growing at the moment? What is going to be the ‘AI’ of 2022 that everyone is asking for? Here, in no particular order are a tech recruiters top three predicted trends;
MLOps
This is not necessarily a new trend, but one that is booming at the moment. It was the worst-kept secret in Data Science that 99% of models never made it to production and actually added tangible business value. With the rise of specific, focussed, Machine Learning Engineers and the advance of new technologies like MLFlow and Kubeflow companies. Businesses are now devoted to building a mature infrastructure that can support models at volume and scale.
It is becoming its own industry to build platforms that support Data Scientists, monitoring and re-training models is fast becoming an essential part of the pipeline. Interestingly, there are now tech startups in the Netherlands like UbiOps or Cubonacci (now acquired by Databricks) designed to help this process and develop new tools to assist the MLOps space.
Currently, dedicated MLOps roles are generally found in larger companies with bigger budgets. However, this doesn’t mean it’s not incorporated into roles in smaller organisations. It’s been found it’s now up to Data Engineers to take on this responsibility (if they didn’t have enough already!).
Either way, it’s an exciting and necessary field so get stuck in!
Data Platform Engineer
This role is a variation on the ‘typical’ Data Engineer profile that you see these days. It incorporates aspects of cloud engineering and software engineering, moving away from traditional data warehousing and pipeline development. As companies’ requirements for data grow, and strategically they move towards a self-serve mentality (every team having a Tableau user for example), the need for a singular data platform grows.
This role is geared towards someone who enjoys various aspects of programming, and typically sees more technologies used along the lines of Terraform, CloudFormation, Kubernetes, and general knowledge of Infrastructure as Code. Arguably this continues the trend of Data Engineers needing to know more and more about DevOps tooling as infrastructure requirements across the organisation are ever more entwined.
The Self-Starter
Companies are starting at earlier stages to incorporate data into their business. Data as an entity started with one ‘specialist’ that cover all aspects of engineering and analytics, before splitting into multiple sections and subsections. However, it’s clear that in this market you have an advantage if you are knowledgable across multiple areas (or may even call yourself a ‘Fullstack Data Scientist’…).
In particular, having this know-how can benefit you in a startup that is beginning to incorporate data. At the onset, you will have to do more engineering, setting up a datawarehouse and basic infrastructure, before moving onto reporting and dashboarding, before getting stuck into the machine learning side of things.
If you choose to go down this route then you have an accelerated path to becoming a Lead and/or Manager. You get to understand the business domain quickly, make the important strategic legacy decisions and you can pick a lot of the low hanging fruit that makes you look great in a CTO’s eyes.
This choice is not for everyone but the levels of impact you have cannot be duplicated in a larger or corporate organisation.
Summary
The data field is only growing, and it’s clear there will be multiple avenues to go down or specialise in over the coming years. These are just three but even within Data Science you could go towards roles focussed on NLP, Computer Vision, Deep Learning, Recommender Systems, Time-series and more.
My advice is usually to stay updated on what technologies are being asked for in job descriptions. Specifically what to focus on? It’s all about the cloud platforms. They’re the fastest moving organisations and releasing new tools quicker than anyone else, so stay on top of your AWS, GCP or Azure knowledge.